Hey guys, welcome to Twelve-Tone! Today, we're going to talk about Twelve-Tone Matrices. And if you stick around till the end, we'll have some tips on turning a row into an actual composition. Like a lot of serialism, this gets a little mathy, but don't worry, it doesn't really go beyond basic arithmetic. On that note, let's talk about notation. Up until now, we've been using standard note names with letters and accidentals. But those names are derived from tonal systems. We use seven letters because our scales have seven notes, and accidentals signify deviations from those scales. However, in twelve-tone music, every note is equal. So when we get deep into it, those names stop being useful. Instead, we just use the numbers zero to eleven, signifying how many half steps above C the note is. So zero is C, 1 is C sharp or D flat, 2 is D, and so on. Once you get to 12, you're back at C, so we reset to zero and continue up again. This system lets us easily visualize intervals, which is more important here than whether a note is sharp or flat. This is just for composition, though. When you're transcribing a piece to be played, you should switch back to regular notation. That's way easier to read. With that in mind, let's talk about the matrix. This is a useful tool for visualizing the structures available to you, and it works like this: you start with a 12 by 12 grid. In the top line, you write your prime rows, starting on C and then going down. In the first column, you write your inversion. A handy trick to find this is to take each number in your prime row and subtract it from 12. So, this 4 inverts...
Award-winning PDF software
Far matrix Form: What You Should Know
B), Contract Language. FAR 6.304(e),”Contracts in Support of the Acquisition and Support of Major Systems,” Federal Acquisition Regulation (Fed. Acquisition Reg. 6, 303). FEDERAL Regulations: 5 C.F.R. Part 2650, Contracts In Support of The Acquisition Of Major Systems. FAR 52.122f,”Use Of Information. (f)(5),”Federal Acquisition Regulations.” CASPAR.7.14 (7) Information in Contract, Clause, or Ration Number Matrix CASPAR.2(4).4. “Contracting Officer's Responsibility to Prevent Contract Deficiencies.” RANGE OF REFERENCE AND OTHER DEFINITIONS A reference matrix is found in FAR 52.301 — “Use of Contract Language.” It describes the types of language that contract provisions and clauses must use in contract documents. The language of clause language and contract provisions must use terms that refer to the contracting activity or agencies, the scope of the contract, the item to be acquired, the nature of the item or service, the quantity to be acquired, and the class to which the item or service is to be assigned. It must make it clear that the language of the contract provisions or clauses is to be used by the contracting activity only, as it was in the prior agreement. The contracting agency or its designee must ensure that the language follows the contract clause definitions. It must include this statement at the top of the contract language: “The contracting activity is responsible for providing the language required to comply with this contract clause” — the contracting officials will be responsible for creating the Contract Defined Language for the entire contract. A reference matrix may be used when: (1) The contracting function may not use standard contract language. Examples to include in the definition of “standard contract language” are language that is repetitive or overly general, or language that excludes the purpose for which the item or service is purchased. The term “must” must be provided in a reference matrix, except as noted in FAR 52.201.1(3)(a) — “All clause terms requiring prior approval to be valid contracts are to be contained in Contracts in Support of the Acquisition and Support of Major Systems.” The language of “must” should be limited to “must” and “must not.
Online solutions help you to manage your record administration along with raise the efficiency of the workflows. Stick to the fast guide to do FaR Clause 52 212-3, steer clear of blunders along with furnish it in a timely manner:
How to complete any FaR Clause 52 212-3 online: - On the site with all the document, click on Begin immediately along with complete for the editor.
- Use your indications to submit established track record areas.
- Add your own info and speak to data.
- Make sure that you enter correct details and numbers throughout suitable areas.
- Very carefully confirm the content of the form as well as grammar along with punctuational.
- Navigate to Support area when you have questions or perhaps handle our assistance team.
- Place an electronic digital unique in your FaR Clause 52 212-3 by using Sign Device.
- After the form is fully gone, media Completed.
- Deliver the particular prepared document by way of electronic mail or facsimile, art print it out or perhaps reduce the gadget.
PDF editor permits you to help make changes to your FaR Clause 52 212-3 from the internet connected gadget, personalize it based on your requirements, indicator this in electronic format and also disperse differently.
Video instructions and help with filling out and completing Far matrix
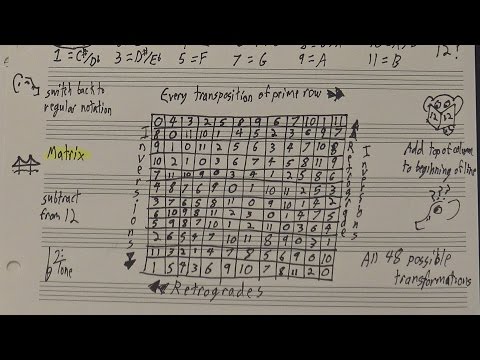